Despite lookalike modeling being a tried-and-true audience segmentation strategy, over 25% of advertisers remain confused about what it is and its significance when it comes to targeting ads online.
As a trusted data management company, we explain what lookalike modeling is and how it can help advertisers discover target audiences based on marketing insights from existing audiences in this guide.
What is lookalike modeling?
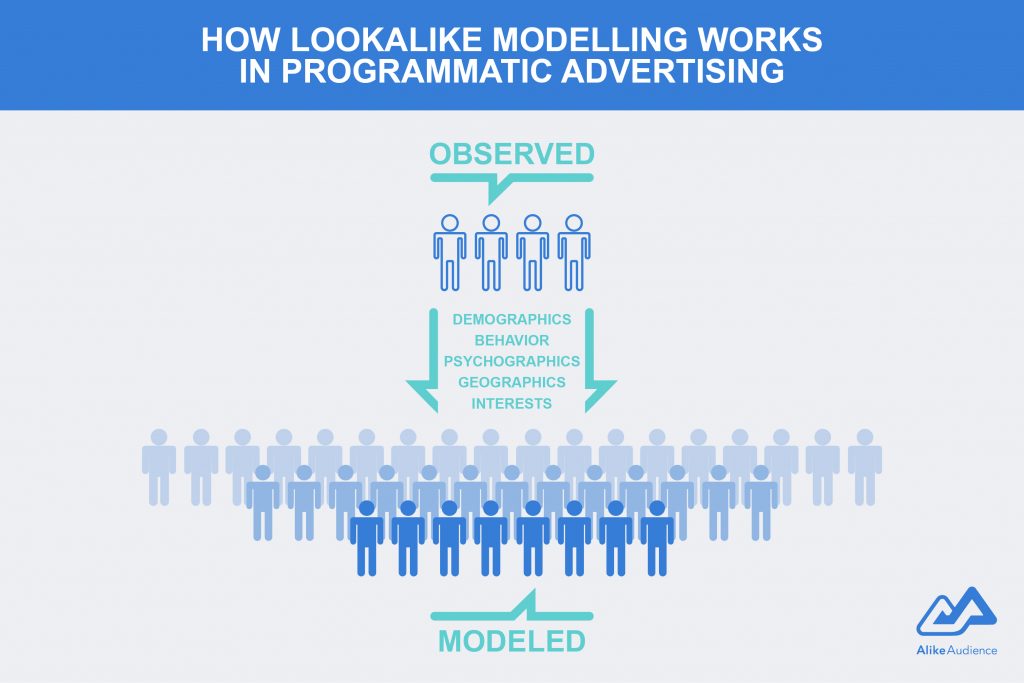
Lookalike modeling is a machine learning technique for identifying new customers that look and behave like your current best customers based on similar behavioral and demographic attributes.
This modeling process involves taking a ‘seed audience’, or your pre-defined best customers, identifying key characteristics that they have in common, and using this information to find new customers that fit those characteristics.
For instance, let’s say you run an e-commerce brand and you’ve defined your best customers, or your “seed pool”, as those who:
• Spend over $100 on average
• Are aged between 20-35 years old
• Live in midwest America
You can find this data through online profiles and behavioral data from your own customer database. Then, you’ll compare this data against third-party consumer data on the open internet that contains these same attributes. We explain this process in detail in the later sections.
Once you get a match, you can target this new prospect that fits this profile on your ad campaigns. Prospects found in this way are much more likely to purchase your product or service than the average customer.
But the good news is, you don’t have to do all this yourself. You can partner with a data vendor, like AlikeAudience, which offers audience segments based on lookalike models. Simply find our audience segments on any demand-side platform of your choice and plug them into your programmatic ad campaign.
Lookalike modeling is an established and well-proven strategy for audience targeting. But how can digital advertisers benefit from it?
Read more: Five Insider Programmatic Advertising Strategies for Digital Marketers
What are the benefits of lookalike modeling for digital marketers?
As marketers, there are a number of ways you and your team can benefit from a lookalike modeling strategy. Here’s how:
• Better understand your existing and potential customers: Lookalike modeling allows you to understand your customers and their journey and behaviors better. Such insights can help optimize your current marketing strategies and future campaigns.
• Improves lead generation: With lookalike modeling, you can easily and efficiently identify potential high-value audiences by just analyzing your existing customer base.
• Increases conversion rates: With lookalike modeling generating high-quality leads, you can expect your click-through rates (CTR) and potential conversions to increase as well. This can, in turn, boost profits and grow your business as a whole.
• Provides more accurate audience targeting: Through machine learning, marketers can analyze and compare data on existing customers and audiences from the wider web, finding the significant and most accurate traits of your best customers in the process.
• Makes for efficient ad spending: Since you’re only targeting audiences that share similar traits with your existing customers, your business can focus on groups most likely to convert.
But how does lookalike modeling itself work? Find out below.
How does lookalike targeting work?
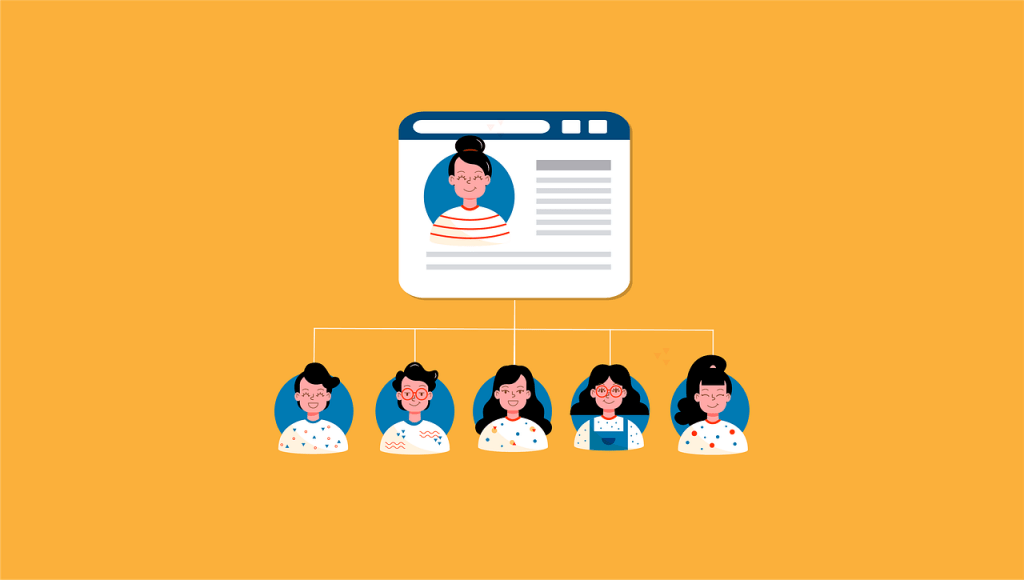
Advertisers carrying out lookalike modeling typically do so on data management platforms (DMPs), as they provide comprehensive tools and resources needed to facilitate the process. Some demand-side platforms (DSPs) also offer services to create lookalike models.
While you can create lookalike audiences on both DMPs and DSPs, the results may differ slightly depending on the type of platform you decide to use.
Lookalike modeling involves a combination of data, machine learning, and mathematical algorithms. When using a DMP, the process itself is comprised of three main steps:
- 1. Collecting data: In order to generate accurate results, different sets of data – such as first-, second-, and third-party data, must be collected from both online and offline sources. Remember – the more data available to you, the more accurate your lookalike audience will be.
- 2. Defining the attributes and behaviors of valuable customers: Once the data is collected, outline the attributes and behaviors of your most valuable customers or seed audience. This could include specific datasets like purchase history, location, frequency and quantity of purchase, or browsing history.
- The attributes and behaviors of your customers should be well-defined in lookalike modeling. The more attributes you identify, the higher your chance of finding high-value customers is.
Bear in mind – even though keeping your seed audience parameters narrow helps locate people most likely to convert, it can also decrease the size of your lookalike audiences. The more attributes you select, the more potential customers will filter out, so choose only the most important first.
3. Extending the audience based on the lookalike model: The final step involves applying mathematical algorithms to find potential audience profiles with similar characteristics that match.
With the help of machine-based predictive modeling, the DMP or DSP analyzes the seed audience and applies proprietary algorithms to the data you collected. User profiles matching the seed audience are then identified, after which you can indicate similarities in the data.
Through lookalike modeling, you can easily pinpoint higher-performing target audiences that you may have been unable to identify beforehand.
AlikeAudience is here to help you find your most profitable customers. Find our lookalike audience segments below.
How does AlikeAudience use lookalike modeling for audience targeting?
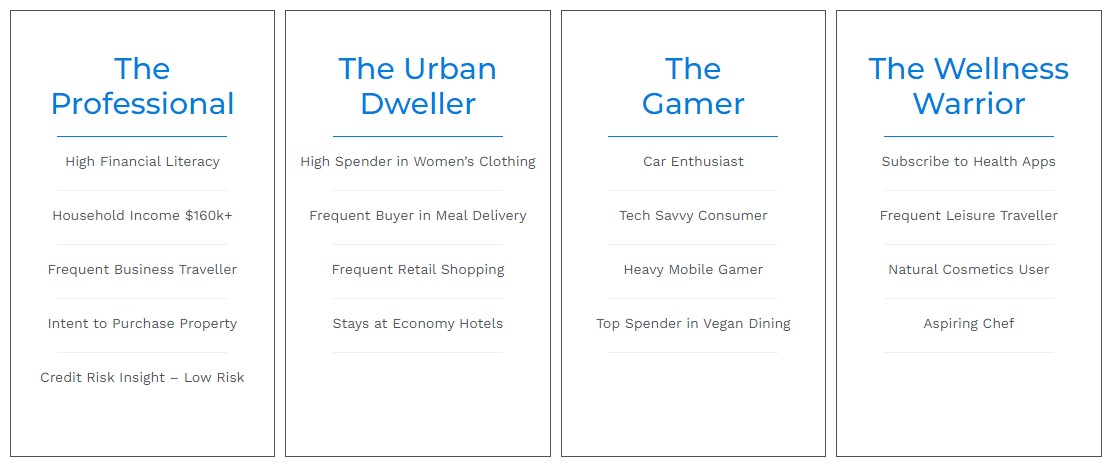
AlikeAudience uses lookalike modeling to create audience segments that are as scalable as possible so you can reach millions of your best customers. The figure above illustrates some of the customer profiles we have.
To build these profiles, we first select attributes to be our seed data (for example, attribute A, B, or C) based on 1.1bn mobile signals and credit card transaction data. We then check for any common attributes within this seed data (for example, they are also common in attributes E, F, and G). Then, we include Attribute E, F, and G in our data. This makes our audience segments scalable.
AlikeAudience uses lookalike modeling to create these types of audience segments:
• Interest: Our interest segments are more persistent because we use mobile attributes to create them. We look at app downloads and install and usage frequency, which is much stronger data signals.
• In-market: In-market segments are also known as purchase intent segments. The categories here are more specific since we look into data observations such as the frequency of engagement with apps and any post-install activities.
• Demographic: These are one of our most widely used products. In some categories of our data products such as age and gender, we use deterministic attributes as its seed audience.
• Reach: This is our latest offer primarily targeted for upper-funnel purposes.
Wrapping Up
Lookalike modeling is a tried and tested advertising strategy that offers big wins for brands looking to grow their business and customer base.
Whether you’re targeting professional mountaineers in their 30s or frequent shoppers of specialized products, partner with a trusted, accurate, and global data management provider like AlikeAudience to hyper-target niche audiences for high ROI campaigns.
With over 7,000 custom audience segments available at AlikeAudience, you can capture high-quality and valuable audiences through on-target demographics, B2B segments, purchase intent from transaction data spending, and mobile app interests with ease.
Find us on your favorite DSP or contact our data strategist for custom solutions. Sign up for our email newsletter for regular ad tech insights!